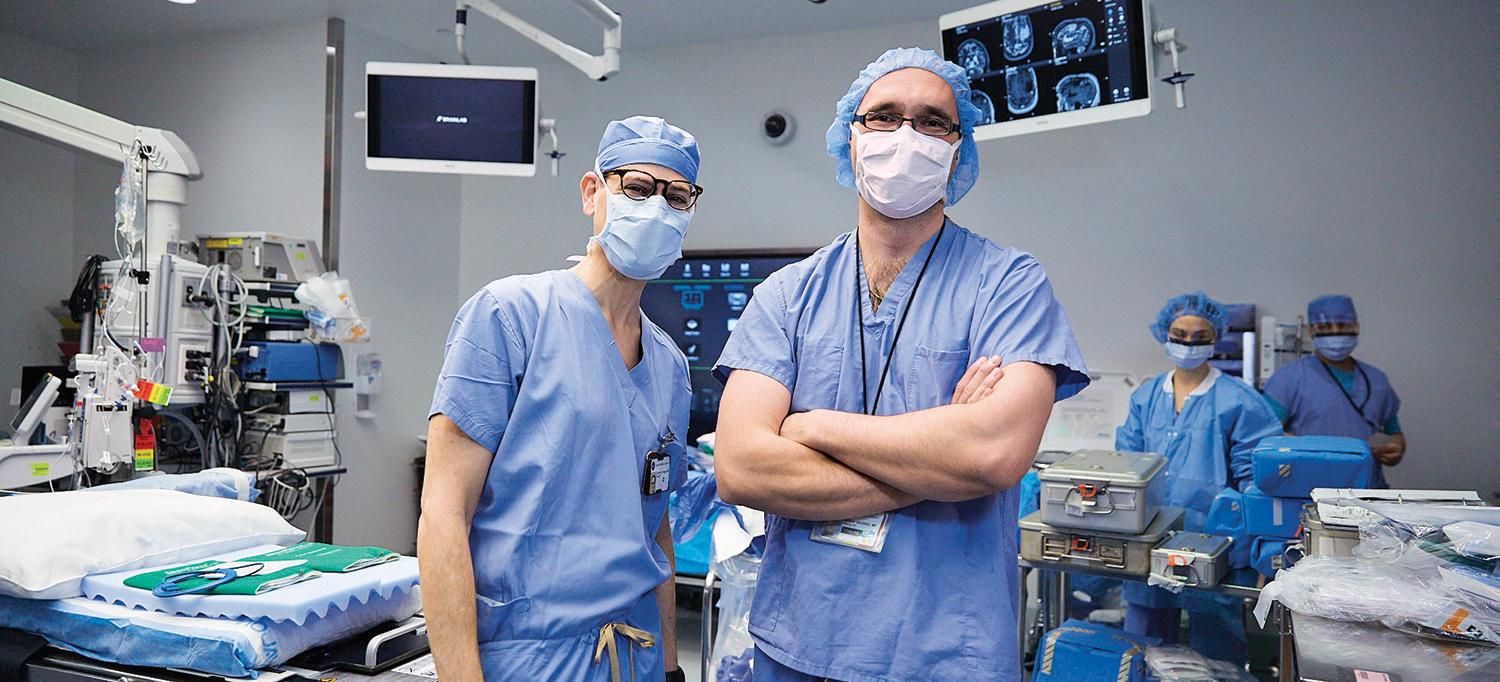
In the operating room, neurosurgeon Dr. Daniel Orringer and neuropathologist Dr. Matija Snuderl employ an imaging system they developed that eliminates the time a patient remains on the operating table while surgeons await pathology results, reducing the risk of infection or complications.
Photo: Jonathan Kozowyk
Observing his first brain surgery as a medical student in 2001, Daniel A. Orringer, MD, felt two emotions in equal measure: fascination and fear. Before him was a neurosurgeon with full command of his instruments. Dr. Orringer looked on as the physician artfully and methodically worked his way through delicate brain tissue. Yet even this master surgeon could not escape the inevitable moment when he would encounter the borders of the deadly tumor blending into healthy tissue like a patch of watercolor.
Save for some form of super, magical vision, it seemed to Dr. Orringer, it would be virtually impossible to know when and where to stop cutting. “I was surprised how much guesswork was required of the surgeon to estimate the boundaries of the tumor,” recalls Dr. Orringer, who joined NYU Langone Health in 2019 as an associate professor in the Department of Neurosurgery at NYU Grossman School of Medicine.
That guesswork defines a central challenge of brain surgery. Cut too little and the residual tumor cells will reboot and kill the patient. Cut too much and critical brain functions could be irreversibly damaged. The dilemma persists even as new technologies have transformed the field of neurosurgery. The advent of magnetic resonance imaging (MRI) in the late 1970s meant tumors could be visualized in advance of an operation; microsurgery has enhanced precision in the operating room; and cancer genetics have improved diagnostics and treatment.
Yet, for some of the most common types of brain cancer, such as gliomas, patients fare no better today than they did in the 1970s. While they are diagnosed more accurately and receive better treatments, their long-term survival prospects have hardly budged. Those with the most aggressive cancers, glioblastomas, may not live a year beyond their surgery.
“Although he’s a neurosurgeon and I’m a neuropathologist, we’re both innovators at heart and driven to solve problems.”—Matija Snuderl, MD, Clinical Associate Professor of Pathology and Director of Molecular Pathology Services
For Dr. Orringer, it seemed clear that those dismal odds would remain unchanged until surgeons had better tools to discern between deadly and healthy tissue in the operating room, while they still had an opportunity to make a difference. “We know that the surgical outcome is one of the most important predictors of patient survival for brain tumor patients,” Dr. Orringer says. “It also happens to be something we can control and improve, unlike how aggressive the tumor is or what part of the brain is involved.”
Indeed, studies show that in up to three-quarters of patients with brain cancer, portions of the tumor that could be safely removed are left behind, simply because the surgeon cannot see them. Without visual certainty, the risk of removing precious healthy tissue that could be involved in speech, memory, movement, or virtually any other important function of the brain is simply too high to cut beyond the known boundaries.
Leveraging Artificial Intelligence to Speed Diagnosis
The gravity of the problem put Dr. Orringer on a career-long path to find a solution, taking him to physicists and their advanced optics, pathologists and their novel genetic methods, engineers, and artificial intelligence (AI) experts. Eighteen years later, he has played a pivotal role in advancing a radically new kind of imaging system, now employed at the Brain and Spine Tumor Center at NYU Langone’s Perlmutter Cancer Center, that helps brain surgeons cut with more confidence. It’s called stimulated Raman histology, or SRH, a method that distinguishes tumor regions, rich in protein and DNA, from normal lipid-rich brain tissue, creating contrasted images akin to conventional histology slides.
The technology is based on an old technique, Raman spectroscopy, used in chemistry since the 1920s, that involves shining a laser beam at a sample. The unique vibrational properties of different molecules change the optical properties of the laser, helping to create an image of the sample’s structure. While powerful at capturing details at submicron resolution, Raman spectroscopy had always been too slow to be of any use in urgent clinical settings. But in 2008, Harvard University scientists had made a breakthrough, fortifying the signal over a million times. “Suddenly, instead of taking all day to acquire an image, it could be done at video rate in a fraction of a second with much higher resolution,” Dr. Orringer says. He set out to adapt the technique for brain tissue.
Since then, Dr. Orringer and team have published a landmark paper in Nature Biomedical Engineering showing that pathologists could just as readily distinguish between cancerous and healthy tissue using the SRH images made in the operating room compared to the conventional ones created in the lab. The system works in concert with a powerful new diagnostic technique that leverages AI to distinguish among tumor types in less than 2 minutes, compared to the 20 to 30 minutes it typically takes human pathologists. The speed of diagnosis is a game changer, eliminating the time that a patient remains on the operating table while surgeons await lab results—a dangerous gap that increases the odds of infection or complications.
“If we have a patient with a tumor of unknown etiology, for example, we might not know whether it’s glioblastoma or lymphoma—two tumors with very different treatments,” Dr. Orringer says. “Making the distinction in the operating room is extremely important.”
Housed in a metal box about the size of a minifridge and mounted on wheels, the technology, which is now available to all NYU Langone Health patients with brain tumors, can be rolled into any operating room to provide a surgeon with near real-time analysis of a tissue sample. “Surgical decision-making is like operating brake and gas pedals,” Dr. Orringer says. “We are taking the guesswork out of the picture by allowing the surgeon to interrogate the tissue on a microscopic level and use imaging data to inform surgical strategy.”
Using Artificial Intelligence to Determine Tumor Type
Brain cancer is a misleading term for what turns out to be a group of more than 120 distinct types of tumors, whose molecular diversity has only recently been recognized thanks to new molecular-profiling technologies. Today, brain tumors are no longer classified based on their histological features alone, but on their molecular signatures, which can determine how the tumors behave. Whether tissue samples are obtained using SRH or conventional methods, they must be analyzed by a specialized pathologist to determine which treatment approach is best for the tumor. “These images are quite complex,” Dr. Orringer says. For that analysis, he has Matija Snuderl, MD, clinical associate professor in the Department of Pathology at NYU Grossman School of Medicine and director of molecular pathology services.
The two physicians were connected through a mutual friend in 2014, when Dr. Orringer was looking for a neuropathologist to independently evaluate the clinical value of the early SRH images. “We immediately hit it off,” Dr. Snuderl recalls. “Although he’s a neurosurgeon and I’m a neuropathologist, we’re both innovators at heart and driven to solve problems.”
When Dr. Snuderl entered medical school, he thought he’d become a pediatric cardiac surgeon. “Then I realized that pathologists are actually behind the steering wheel of medicine,” he says. “Surgeons, oncologists, neurologists, they all rely on our diagnosis, and if the diagnosis is incorrect, everything else will be wrong.”
Noting the importance of diagnosing cancers at a molecular level, Dr. Snuderl has been developing novel tests to reveal a tumor’s unique molecular signature. One such test is a brain cancer–profiling method that goes beyond gene mutations and considers DNA methylation profiles—that is, the epigenetic alterations that can arise from those mutations. This extra layer of information can show how two tumors that look identical under the microscope may in fact behave quite differently, depending on their environment. How fast a tumor grows or how well it responds to medication could vary significantly, subject to its epigenetic profile, and this, in turn, impacts the neurosurgical approach.
“The neurosurgeon can decide to be more or less aggressive based on what we tell them,” he says. For that to actually work, however, the neurosurgeon would need the information during the operation. Molecular tests, though, take two weeks to carry out.
Dr. Snuderl and Dr. Orringer reasoned that they could possibly solve the speed problem by training an AI algorithm to diagnose a tumor based on what it looks like on SRH images taken during surgery. “A real innovation is bringing that diagnostic information to the operating room in real time, where the surgeon can act on it,” Dr. Orringer says.
In a recent study published in Nature Medicine, the team tested the AI technology on the 10 most common categories of brain tumors. They trained an algorithm on SRH images of 415 patients with brain cancer and then tested it against expert pathologists evaluating samples from a separate group of 278 patients having surgery. Both the algorithm and neuropathologists correctly diagnosed 94 percent of the tumors. Notably, the mistakes made by the algorithm and neuropathologists were different. If the methods had been combined, diagnostic accuracy would have approached 100 percent. “If the algorithm was in the hands of the pathologist, it would have been possible to avoid a single misdiagnosis,” Dr. Orringer says.
“We can essentially deliver the capacity to detect and diagnose brain tumors with accuracy traditionally found only in the most well-resourced centers, and with unprecedented speed,” Dr. Snuderl says. “It stands to reason that, particularly in the management of difficult cases, this will have a big impact on patients.”