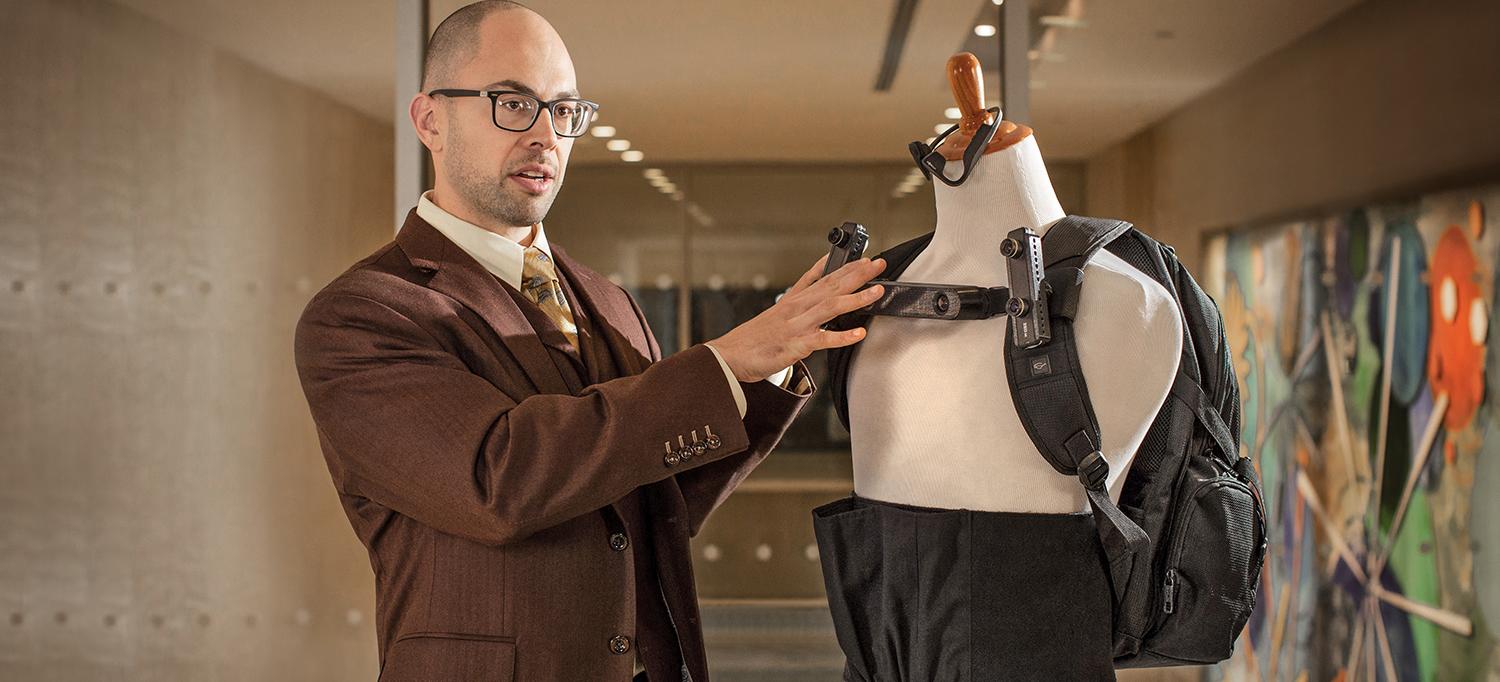
Dr. JohnRoss Rizzo’s lab is developing technology that helps people with conditions such as blindness and stroke to interact physically with their environment.
Photo: NYU Langone Staff
Recent advances in miniaturized sensors and actuators—as well as artificial intelligence—have broadened horizons for assistive and rehabilitative technologies. The laboratory of JohnRoss Rizzo, MD, assistant professor in the Departments of Rehabilitation Medicine and Neurology, is leveraging these innovations to help patients with conditions such as blindness and stroke, enhancing their ability to interact physically with their environment.
Step-by-Step Guidance for Visually Challenged Pedestrians
Dr. Rizzo’s focus is driven, in part, by his own experience as a patient with choroideremia—an inherited, progressive eye disorder that has left him legally blind. His team at Rusk Rehabilitation, in partnership with NYU Tandon School of Engineering, is developing advanced wearable devices to provide visually impaired pedestrians with step-by-step navigational instructions and obstacle warnings. “Most wearables are designed to provide interoceptive information, like heart rate or sleep quality,” Dr. Rizzo explains. “Our devices focus on the wearer’s exteroceptive needs. We’re working to connect the quantified self with the quantified environment.”
These devices, currently in prototype form, are based on technology similar to that used in self-driving cars. The user wears a backpack, a waist belt, and headphones. The belt and shoulder straps are fitted with specialized cameras as well as infrared and ultrasound sensors, which transmit data to a microcomputer carried in the backpack. Visual imagery is processed by deep-learning software that is trained to recognize objects and faces as well as the user’s gestures, and to calculate the best route to a designated terminus. At the entrance to a supermarket, for example, a synthesized voice identifies each landmark (“door, table, shopping carts”) as the user sweeps a pointing finger from left to right. The user then uses gestures to indicate which object they wish to engage with, and the voice offers detailed guidance toward it. Haptic motors in the belt and straps provide error correction.
In April 2019, a team led by Dr. Rizzo presented at the Computer Vision Conference in Las Vegas on one of the lab’s projects: Cross-Safe, a computer vision–based approach to making intersection-related pedestrian signals accessible for the visually impaired. Conceived as part of a larger wearable device, Cross-Safe uses a compact processing unit programmed with a specialized algorithm to enable identification and interpretation of crosswalk signals, and to provide situational as well as spatial guidance. A custom image library was built and developed to train, validate, and test the team’s methodology on actual traffic intersections. Preliminary experimental results, to be published in 2020 in Advances in Computer Vision, showed a 96 percent accuracy rate in detecting and recognizing red and green pedestrian signals across New York City.
Helping Stroke Patients Regain Eye–Hand Coordination
For many stroke patients, seeing an object isn’t the problem; reaching for it is. Beyond any underlying sensorimotor deficits, as Dr. Rizzo’s past research has helped demonstrate, stroke may impair eye–hand coordination by disrupting the cycle of feedforward predictions and feedback-based corrective mechanisms that normally link visual planning and limb movement. Existing rehabilitation techniques have limited success in restoring this delicate relationship. “Patients often hit plateaus in terms of recovery,” he observes. “We’re developing therapies designed to break through those plateaus and further boost function.”
In a study published in June 2019 in Progress in Brain Research, Dr. Rizzo and his colleagues pursued that goal using a computer game–like system that provided extrinsic feedback to correct reaching errors. Although such approaches have previously been explored in eye–hand re-coordination studies, they have targeted only the hand. This study was the first to test a biofeedback-based technique aimed at retraining the eyes as well.
Participants included 13 patients with a history of middle cerebral artery ischemic stroke and 17 neurologically sound controls. Dr. Rizzo’s team used a headset fitted with miniature cameras that tracked each subject’s eye movements. A sensor attached to the index finger tracked hand movements across a table. To assess potential learning effects (secondary to the feedback focused on ocular motor errors), subjects participated in two trial blocks involving a prosaccade look-and-reach task.
Subjects were instructed to move their eyes and finger as quickly as possible to follow a small white circle on a computer screen. In the first experiment, they received on-screen feedback showing any discrepancy between the final location of the circle and that of the finger. In the second experiment, the feedback also included any discrepancy between the location of the circle and that of the subject’s gaze. In each experiment, controls participated in one session; stroke patients completed up to two sessions, one for each arm (if they were capable). Each session consisted of 152 reaches.
In the first experiment, the primary saccade produced by stroke participants consistently occurred earlier than in healthy participants, with finger movement lagging behind. Over the course of the second experiment, however, stroke patients significantly improved their performance—reducing errors in the timing of saccades and the accuracy of reach in both the more- and less-affected arms. (Non-stroke patients, paradoxically, grew slightly less coordinated when given feedback including ocular errors.) “We believe visual feedback through extrinsic spatial prompting served here has the potential to improve eye movement accuracy,” Dr. Rizzo and his co-authors wrote. Although further studies are needed to optimize therapeutic outcomes, these results indicate that extrinsic feedback, in appropriate doses, may be a valuable tool for enhancing ocular motor capabilities in the setting of eye–hand coordination for stroke rehabilitation.